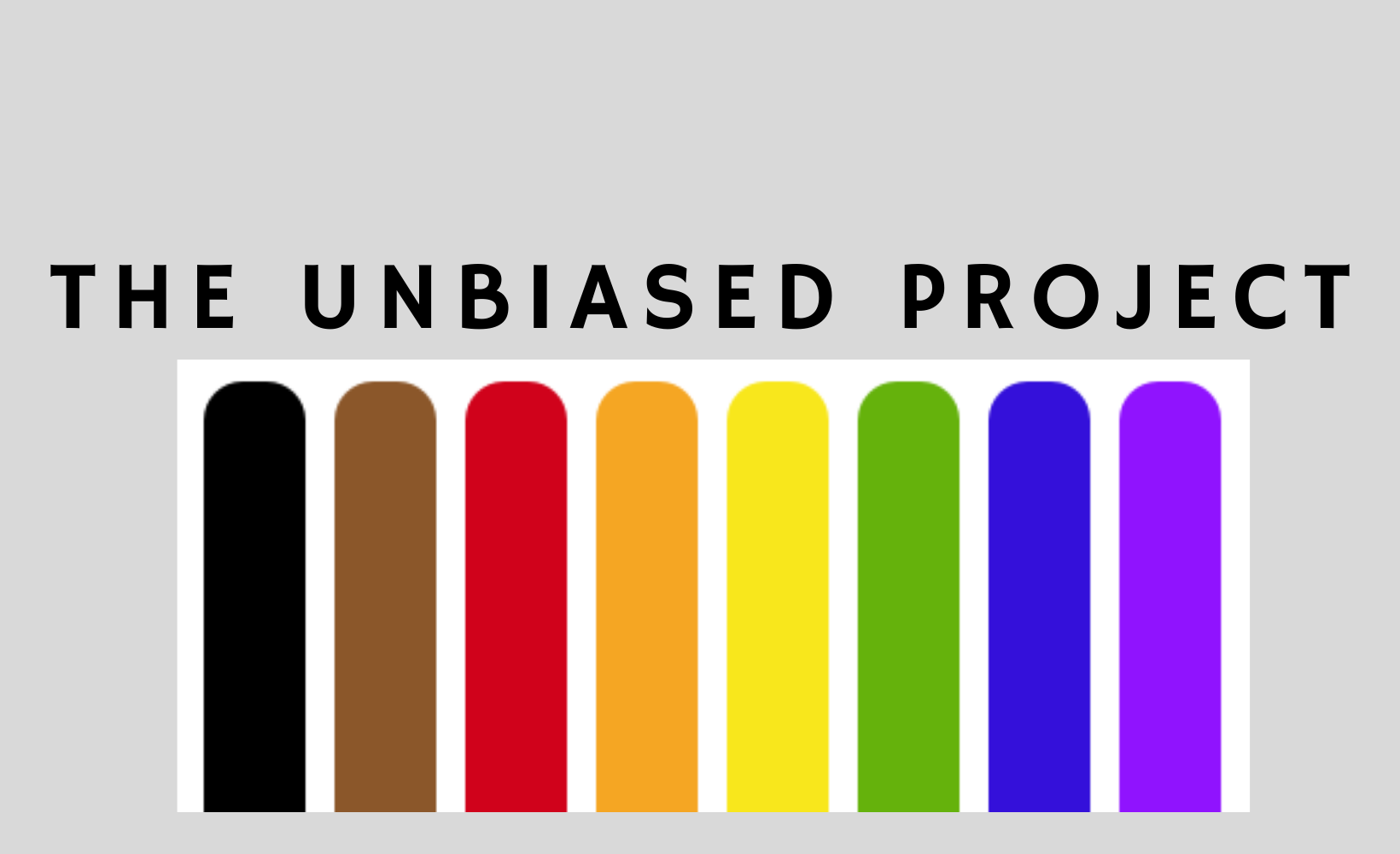
A joint research project through UCSD and UW that investigates implicit bias in patient-physician interactions.
Overview.
By utilizing affect coding and social signal processing coding, we hope to uncover the behaviors and moments that showcase a provider being unconsciously biased towards their patient, in hopes of utilizing this data to create mechanisms that can increase awareness and reduce occurrences of bias in patient-physician interactions.
Background.
Unequivocal power imbalances. Demoralization of patients. Engrained hierarchy. This is what centuries of perpetrating inequality has led our healthcare system to. This same system has continually praised physicians and led patients into docile roles, where they feel powerless, hopeless, and disregarded. This ignorance has perpetuated many issues within the healthcare industry and it is time for a culture shift, where the needs of the patients are met first.
The UnBIASED Project utilizes a combination of affect coding and social signal processing coding to understand the role of implicit bias in patient-physician interactions. By analyzing both communication and non-communication channels we hope to discover behavior patterns that reveal bias. Through the utilization of this coded data, we hope to develop mechanisms that can raise awareness about implicit bias and decrease the occurrence of biased interactions.
Problem.
The strong patient-physician hierarchy that has perpetrated for centuries within the healthcare system has created an uneven power dynamic, forcing physicians into dominant roles. How can we shift the culture and create a more equitable healthcare system for patients?
Significance.
The healthcare system has a very discriminatory history that has carried over into present day through more silent, subconscious channels. Implicit bias in physicians has greatly impacted the healthcare outcomes of patients and has perpetuated a system of oppression.
This project can bring about important and much needed changes within the healthcare industry. Patients have been complacent for too long and it is time to empower them so that they can receive the care that they need and the care that they deserve.
This project can also raise awareness about perpetuating issues within the healthcare system that have plagued society for centuries.
It is time for a culture shift, where we put the needs of the patient first.
Coding Tools.
The coding is divided into two categories: emotional valence and behavioral content. The combination will be able to provide us with a robust understanding of the entire interaction. Below are the interfaces that we will be utilizing to code the interactions, the first being used for the emotional valence coding (RIAS GAR Coding) and the second for the behavioral content coding (behavioral orientation).
Thin Slice Coder
The application we are using to conduct the thin slice coding can be seen in the image below. It includes a video player as well as sections with drop-down menus for coders to efficiently input ratings for each thin-slice of the Global Affect Rating. After rating for a thin slice is completed, coders will download individual CSV files of the slices for additional analysis.
BORIS
BORIS or Behavioral Observation Research Interactive Software was used for the Social Signal Processing coding. Overall four different modalities were coded using BORIS: Body Orientation, Head (Gaze) and Emotion, Hands and Arms, and Verbal. Specific modifiers were used to indicate when each behavior was performed and by which subject (Physician, Other Provider, Patient, or Patient Companion) during the interaction. Utilizing literature review findings, an ethogram— an inventory of behaviors of interest— was created for modality that will be coded.
Emotional Valence.
To assess the emotional context within the interactions, we are using the Global Affect Rating Thin Slice Coding approach, which is derived from the Roter Interaction Analysis System (RIAS). The Global Affect Rating is a 1-6 Likert scale rating system that analyzes the emotional context of the patient, provider, patient companion, and medical assistants during the interaction.
We will utilize a thin slice coding approach, meaning that the coders will rate the emotional context of 3-minute subsections of the interaction. By doing this, we can visually see the fluctuations in emotional context throughout the entire visit, therefore providing a robust analysis of the interaction. For the Global Affect Rating portion of the coding, we will utilize only the auditory modality of the pre-recorded patient-provider interactions, so that we are not swayed by the visual or behavioral aspects of the interaction. This way we can fully focus on coding the emotional content of the interaction.
Body Orientation.
Body orientation includes torso orientation, lean, proximity, gesture (shrug, other, etc), and sitting vs standing. These multiple facets will give a robust understanding of the non-verbal communication between the physician, patient, and patient companion. Below is a detailed ethogram of the coding scheme, including specific modifiers.
Lean.
Lean has to do with the angle of the patient or the physician’s torso. Literature has proposed that lean is an important indicator of attention and engagement. We are looking for the physician to be leaning forward and towards the patient, since this is a key sign of attention. This is commonly considered the body position that suggests high engagement. There are four modifiers for lean: forward, backward, towards the other individual, and away from the other individual.
Sitting vs. Standing.
Literature has indicated that sitting versus standing can be an important category to look at, especially considering the juxtaposition between the two body positions. If the patient is sitting, but the physician is standing, this can provide some important insight into the power dynamic between the two participants and their respective roles in the interaction. This can often be a subconscious action that may make the patient feel subordinate and is why this is an important category to code.
Torso Orientation.
When coding the torso, we are looking for both shoulders to be facing the proposed direction. So if one shoulder moves slightly towards a different position, but the other is still in the original position, then it will not count as a torso orientation change. In the torso category, we have six different modifiers: patient companion, computer, physician, patient, clinical staff, and other. These modifiers are used to identify what/who the torso is facing. If the torso is facing a different area not noted in the specific modifiers, mark as other and write a comment indicating what the individual is facing.
Proximity.
For proximity, we are looking for the patient or physician to physically alter their position in the room to get either closer or further away from the other individual. Even if the patient/physician is leaning and getting closer or further away from the other, because they are not physically getting up to change that distance, it does not count as proximity. For proximity, there are three modifiers: closer, further away, and side-by-side. If the patient or physician physically moves closer to the other participant, it must be coded as closer. If they move further away, then mark as further. For side-by-side, we are looking for the physician to get closer to the patient by sitting adjacent to them.
Gesture.
Gesture, in terms of the body, refers to shrugs or any other movement that involves the shoulders moving. A shrug is defined as a movement of the shoulders in the upward direction, where the shoulders move closer to the ears. A shrug is defined as a movement of the shoulders in the upward direction, where the shoulders move closer to the ears. A shrug with just one shoulder should be coded and considered as a shrug. If the patient/physician makes a shrug-like movement or gesture, but context-wise does not make sense to code as a shrug, just code it as “other” and write a comment regarding the context of the movement and detailing what the movement was.
ChronoViz.
Chronoviz is utilized as a data visualization tool to collectively analyze the social signal processing coding completed in BORIS as well as the thin slice affect coding. Through this software, we uploaded CSV files of the coded interactions and were able to separate each modality into its own timeline. Furthermore, each subject had its own color (identifier) to allow for visual identification of the annotations within each timeline (modality). By doing so, we were able to find breakdowns and repairs in the patient-physician interaction by looking for moments of congruence between the social signals. This allows us to look at specific moments of interest for further analysis.